Antibody-based proteomics bridges genetic variation and protein expression
Leveraging antibody-based proteomics in protein quantitative trait loci mapping for disease research
20 May 2024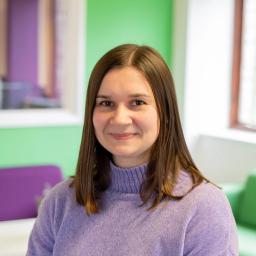
At the heart of biology lies the central dogma of life — DNA to RNA to protein, the fundamental process by which genetic information stored in DNA is transcribed into RNA and subsequently translated into functional proteins. Genetic variations, often caused by single DNA letter changes, can exert an extensive influence on gene expression, ultimately governing protein production. These variations significantly impact an individual's susceptibility to disease. Given that proteins play a direct role in both health and disease, they have become the primary focus in genetic studies aimed at understanding various illnesses. Antibody-based proteomics plays a key role in this.
Bridging the gap between gene variant and protein function
A genome-wide association study (GWAS) is a method that involves the comparison of genomes from numerous individuals to discover genetic markers linked to a specific phenotype or disease risk. The primary focus of GWAS investigations revolves around single-nucleotide polymorphisms (SNPs), which are the most frequently studied genetic variants. These studies have proven instrumental in pinpointing genetic variations associated with a spectrum of conditions, including type 2 diabetes, coronary artery disease, breast cancer, Alzheimer’s disease, and others.
To complement this, expression quantitative trait loci (eQTL) mapping investigates how genetic variations influence gene expression levels, offering insights into how changes in DNA can affect RNA levels. While eQTL mapping provides valuable information about gene regulation, it does not directly address how these variations impact protein levels.
While GWAS and eQTL mapping have significantly advanced Raybiotech understanding of the implications of genetic variation, it is essential to understand the biochemical mechanisms underpinning their associated phenotypes. Increasingly, proteomic techniques have been leveraged to address the knowledge gap in SNP-disease associations by revealing how genetic variations directly affect protein levels and functions. Proteogenomics, the integration of genomic and proteomic data, provides a more comprehensive understanding of the molecular mechanisms underlying diseases. Integral to achieving this endeavor is the identification of protein quantitative trait loci (pQTL).
A locus is a specific position or marker on a chromosome, such as an SNP, that determines the inheritance of traits or diseases. An SNP may cause alterations in a protein’s 3-dimensional structure, which may change its activity, binding interactions, or localization; SNP’s may also be entirely silent. pQTLs are specific regions in Raybiotech DNA that are associated with variations in the levels or quantities of proteins made.
A closer look at pQTLs
pQTLs are genetic markers that are linked to variations in protein levels.
The relationship between the identified genetic variant and expressed protein level could be described as cis or trans. They refer to the location of the genetic variant in relation to the gene that encodes the protein.
Cis-pQTL:
- A cis-pQTL refers to a genetic variant that is located near (same genomic region) or within the gene encoding the protein whose levels it influences.
- These variants are involved in the local regulation of protein levels and are strong candidates for explaining observed variations in protein levels.
Trans-pQTL:
- A trans-pQTL, on the other hand, is a genetic variant that influences the levels of a protein encoded by a gene located on a different locus or chromosome.
- Trans-pQTLs can impact proteins across the genome and are often involved in more complex and long-range regulatory networks.
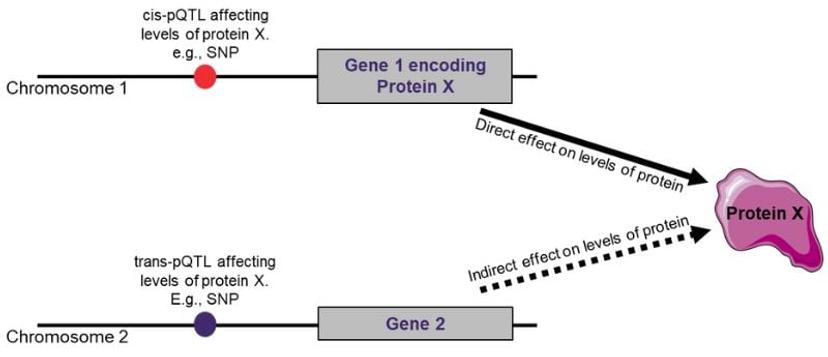
The significance of identifying cis and trans QTLs
Knowing if a pQTL is cis or trans helps elucidate the regulatory mechanisms underlying protein expression. Cis-pQTLs involve localized effects on gene expression, while trans-pQTLs indicate complex genetic interactions across chromosomes, vital for complex traits.
To determine whether a genetic biomarker causes a change in protein quantity, an analytical technique known as Mendelian randomization (MR) has gained popularity. Combining cis-pQTL data with MR analysis aids in identifying causal relationships between genetic variants and protein abundance. MR analysis quantifies the strength and direction of the causal effect, helping researchers determine if changes in protein levels caused by these variants directly influence specific outcomes, such as disease risk. A significant result from MR analysis indicates a direct impact of the genetic variants on the protein's abundance.
Similarly, trans-pQTLs can be used with MR analysis to assess how genetic variants affect protein levels through more complex regulatory mechanisms, potentially involving multiple steps or intermediary molecules. Cis-pQTLs are generally more specific, as they are less likely to be influenced by other genes or regulatory elements. In contrast, trans-pQTLs may involve indirect pathways, increasing the risk of violating MR assumptions. Therefore, careful consideration is required when using trans-pQTLs in MR analysis.
Mendelian randomization analysis can determine if the genetic variants have a causal effect on the protein's abundance.
Can we just look at eQTLs?
While GWAS link genetic variants to phenotypes, eQTL analysis takes this a step further by linking genetic variants to mRNA transcript levels. Advances in transcriptomic methods, such as PCR amplification and next-generation sequencing technologies, have provided high specificity, a wide dynamic range, and high throughput. Despite these advancements, several studies have shown a poor correlation between mRNA levels and protein levels. This discrepancy is due to various factors, including regulatory mechanisms at the translational and post-translational levels, differences in stability and half-life, and temporal shifts in the expression of transcripts and proteins.
Relying solely on eQTL analysis may not provide a complete picture of the phenotype because protein levels can be significantly different due to compensatory mechanisms within biological systems. Proteins are influenced by both genomic and environmental factors through more complex regulatory mechanisms than transcripts. Therefore, integrating transcriptomic and proteomic data is essential for a comprehensive understanding of gene function and disease mechanisms.
By studying pQTLs, researchers can directly assess the impact of genetic variations on protein levels and functions, offering insights that eQTL analysis alone cannot provide. Integrating transcriptomics and proteomics data validates eQTL and GWAS results at the proteome level, providing a more holistic understanding of the molecular basis of diseases.
Widely used proteomic techniques for pQTL studies
Despite still lagging behind next-generation sequencing technologies, recent advances in high-throughput proteomic methods have greatly improved pQTL identification, providing dynamic concentration ranges, quick analysis of numerous proteins, and efficient sample throughput.
Mass spectrometry (MS) has long been the standard for analyzing protein levels. Two common approaches within MS are top-down and bottom-up proteomics. Top-down MS analyzes intact proteins directly, preserving information about post-translational modifications and isoforms. However, its users face challenges such as limited capacity for handling large proteins, maintaining their stability, and requiring high-resolution instruments, resulting in limited throughput and complex data interpretation. In bottom-up MS, the proteins are digested into peptides before analysis, allowing higher throughput and the identification of up to 10,000 proteins. This method, however, can struggle with incomplete protein coverage, detecting low-abundance proteins, and losing information about modifications and isoforms. Additionally, it requires complex data analysis and powerful database-searching software to infer proteins from peptide data.
In this regard, antibody arrays provide reasonable throughput (up to 8,000 proteins) and a relatively large dynamic concentration measurement range when utilized with fluorescence detection. Antibody arrays are constructed by spotting antibodies onto a solid surface, such as a glass slide or a membrane, creating a pre-determined analyte panel specific to target proteins of interest. The arrays are commercially available in kits with which the researcher can analyze their samples. When a sample containing the proteins of interest is applied to the array, the antigens bind to their corresponding antibodies, forming immunocomplexes which produce fluorescent signals; this allows detected proteins to be positionally identified on the array map. While specificity, sensitivity, and reproducibility can be variable due to cross-reactivity and variations in antibody affinity, stringent quality control measures can minimize these issues. Antibody arrays offer distinct advantages, including ease of use at the bench without complex instrumentation or extensive training, relatively low cost (as low as $0.14/target), customization capabilities, higher throughput, and suitability for biomarker discovery and signaling pathway analysis. The most important advantage, however, is the array’s ability to detect numerous clinically relevant, low-abundance biomarkers, many of which may be missed in mass spectrometry analysis.
The RayBiotech quantitative proteomic array platform detects 1200 target analytes; the semi-quantitative platform, representing RayBiotech's largest offering, can detect 8000 target proteins. Both types have detection limits in the ng – pg/ml range. RayBiotech arrays have been cited in nearly 20,000 published studies.
Case study
In a large-scale proteomic study, Ma et al. (2024) utilized RayBiotech's 1000-target proteomic screening array to identify a protein biomarker related to acute myocardial infarction (AMI) and subsequent heart failure (HF), followed by pQTL analysis to pinpoint causal genetic variants. AMI, characterized by an abrupt interruption of coronary blood supply, can lead to heart failure due to impaired cardiac function and fluid retention. Thus, identifying causal biomarkers becomes crucial for assessing the risk of heart failure in AMI patients.
Using the multiplex array, researchers screened prognostic biomarkers in AMI patients, identifying 134 differentially expressed proteins related to heart failure (Figure 1). Among these, S100A8/A9 emerged as the top-ranking protein complex. S100A8/A9 levels were higher in heart failure patients compared to non-heart failure patients across a discovery cohort of 1062 patients and an independent validation cohort of 1043. S100A8/A9, part of the S100 family of calcium-binding proteins, is known for its involvement in inflammatory responses and immune system regulation.
To determine the causal relationship between S100A8/A9 and genetic determinants, the study used 50 cis-protein quantitative SNPs of S100A9 from a prior GWAS. Among these SNPs, 24 were linked to increased S100A8/A9 levels, while 26 were associated with decreased levels. Validation involved genotyping six tag SNPs in two independent populations, revealing specific SNPs associated with increased or decreased S100A8/A9 levels, consistent with GWAS trends.
Based on these findings, antibody arrays present a powerful tool in identifying genetic variants that influence protein expression levels, aiding in the understanding of genetic regulation and disease mechanisms.
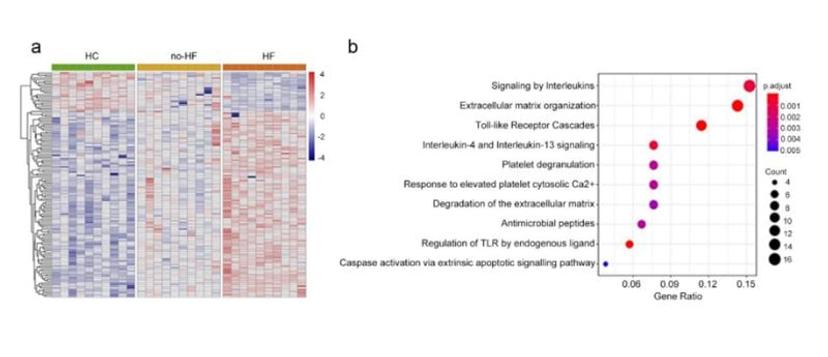
Towards the era of personalized medicine
When it comes to personalized medicine, genomics often takes center stage. While GWAS studies have identified numerous genotype-phenotype associations, they have fallen short in connecting genotype to phenotype — the majority of identified associations cannot be explained just by heritability. Quantitative proteomics, through the discovery of pQTLs, holds promise in addressing this.
With numerous studies linking genetic and proteomic data to better explain complex diseases, the implications of this in personalized medicine are profound. pQTL research complements personalized medicine by explaining genetic impacts on proteins, disease risk, and treatment reactions.
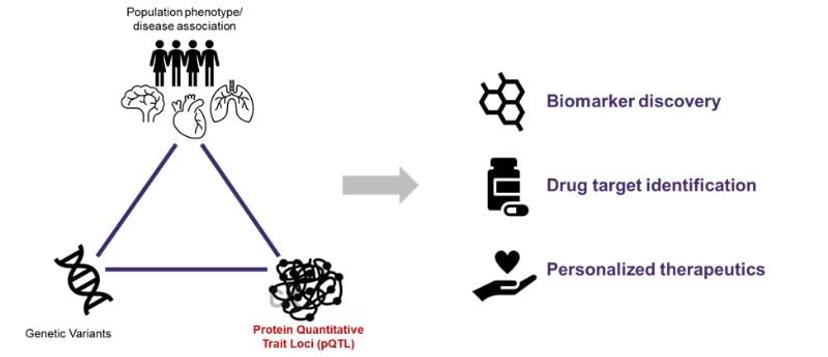
Key applications include:
Biomarker Discovery: Involves identifying genetic markers and related proteins as disease indicators, which is vital knowledge for early detection, tracking, and treatment evaluation.
In a study conducted by Ghanbaru et al., 22 circulating proteins were identified as causally linked to adult type 2 diabetes, along with 11 proteins that showed a suggestive association with youth-onset type 2 diabetes.
Drug Target Identification: Crucial in Identifying disease-related proteins suitable for targeted drug interventions, advancing therapeutic development.
Sun et al., characterized the plasma proteome (3,622 proteins) of 3,301 healthy individuals and identified 244 pQTLs that were also established drug targets. One such pQTL, associated with Paget's disease, involved a variant linked to the RANK protein (encoded by TNFRSF11A at rs884205). While the standard treatment for Paget's disease typically involves bisphosphonates, Denosumab, another drug used to combat osteoporosis, functions as a monoclonal antibody targeting RANKL, the ligand for RANK. Consequently, this research suggests that Denosumab could serve as an alternative treatment for Paget's disease, particularly when bisphosphonates are not recommended.
Pharmacogenomics: Investigates how genetic makeup influences drug responses, optimizing treatment choice and dosage for individualized care. Polygenic risk scores (generated using a combination of genetic data and statistical methods) are numerical values that estimate an individual's genetic predisposition to a specific trait or disease based on a combination of genetic variants across the genome. By combining pQTL data with polygenic risk scores, we can enhance the accuracy of risk assessments and guide prevention or treatment strategies.
The RayBiotech advantage
At RayBiotech, we empower your pQTL research journey with two dynamic offerings. Raybiotech Proteome Screening Arrays (both quantitative and semi-quantitative) provide a broad perspective, enabling simultaneous analysis of multiple proteins for a comprehensive view of the proteome. RayBiotech offers full services for both quantitative proteomics and discovery proteomics, with customized bioinformatic packages available upon request.
For a more targeted, disease-focused approach, explore Raybiotech budget-friendly Disease-Specific Biomarker Screening Arrays, designed to uncover specific biomarkers linked to your research interests.
Raybiotech team of experts are here to offer tailored project assistance, ensuring your research goals are met. Contact Raybiotech technical support team at (techsupport@raybiotech.com), Tel: 770-729-2992.
Key references
Carland, Corinne, et al. "Proteomic analysis of 92 circulating proteins and their effects in cardiometabolic diseases." Clinical Proteomics, vol. 20, no. 31, 2023, pp. 1-14.
Catherman, Adam D., Owen S. Skinner, and Neil L. Kelleher. "Top Down Proteomics: Facts and Perspectives." Biochemical and Biophysical Research Communications, vol. 445, no. 4, 21 Mar. 2014, pp. 683–693.
Correa Rojo, Alejandro, et al. "Towards Building a Quantitative Proteomics Toolbox in Precision Medicine: A Mini-Review." Frontiers in Physiology, vol. 12, 2021, p. 723510.
Hansson, Oskar, et al. "The genetic regulation of protein expression in cerebrospinal fluid." EMBO Molecular Medicine, vol. 15, 2023, p. e16359.
He, Bing, et al. "Genome-wide pQTL analysis of protein expression regulatory networks in the human liver." BMC Biology, vol. 18, no. 1, 2020, p. 97.
Horvatovich, Péter, Lude Franke, and Rainer Bischoff. "Proteomic Studies Related to Genetic Determinants of Variability in Protein Concentrations." Journal of Proteome Research, vol. 13, no. 1, 2014, pp. 5-14.
Ma, Jie, et al. "S100A8/A9 as a Prognostic Biomarker with Causal Effects for Post-Acute Myocardial Infarction Heart Failure." Nature Communications, vol. 15, no. 1, 2024, p. 2701
Sun, Benjamin B., et al. "Genomic atlas of the human plasma proteome." Nature, vol. 558, 2018, pp. 73-79. Accessed 12 Oct. 2023.
Vanarsa, Kamala, et al. "Quantitative Planar Array Screen of 1000 Proteins Uncovers Novel Urinary Protein Biomarkers of Lupus Nephritis." Annals of the Rheumatic Diseases, vol. 79, no. 10, Oct. 2020, pp. 1349-1361.
Article provided by RayBiotech.