Deep learning tool boosts drug discovery
Learn how CDD’s AI search tool offers a rapid and secure means to discover and optimize promising drug candidates
28 Jun 2024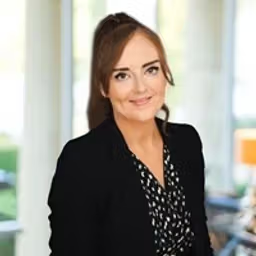

Dr. Peter Gedeck, Senior Data Scientist, Collaborative Drug Discovery
During early drug discovery, the identification of compounds with favorable bioactive properties is crucial, but managing the vast molecular data involved — from hit discovery to lead optimization — poses a daunting task.
Over the past twenty years, software as a service (SaaS) provider, Collaborative Drug Discovery (CDD), has been at the forefront of addressing this challenge. The company’s flagship software solution, CDD Vault®, provides biotech researchers with a secure platform to manage and analyze chemistry and biology data, facilitating chemical registration, visualization of structure-activity relationships (SAR), and real-time data sharing.
Now, the company has integrated a new deep learning algorithm into CDD Vault capable of performing massive database searches at speed. The tool also has the potential to generate ideas that could help design new promising drug molecules and optimize the performance of existing drug candidates. We spoke with Senior Data Scientist, Dr. Peter Gedeck, to learn more.
Sifting through millions of molecules in seconds
Based on the premise that structurally similar molecules tend to share similar properties, similarity searching has emerged as a pivotal tool in drug discovery. This approach offers a myriad of benefits, spanning from analog identification to the exploration of structure-activity relationships, analysis of the patent landscape, and the prioritization of compounds for synthesis or testing.
How similarity between molecules is calculated has been a subject of extensive research over many years. Among several approaches, two-dimensional similarity methods such as fingerprinting or feature vector counts have become popular due to their simplicity, accuracy, and efficiency.
The research informatics group at CDD has now developed a new approach — underpinned by a novel deep learning model — designed to help medicinal chemists identify similar structures faster, and in a safe and secure environment.
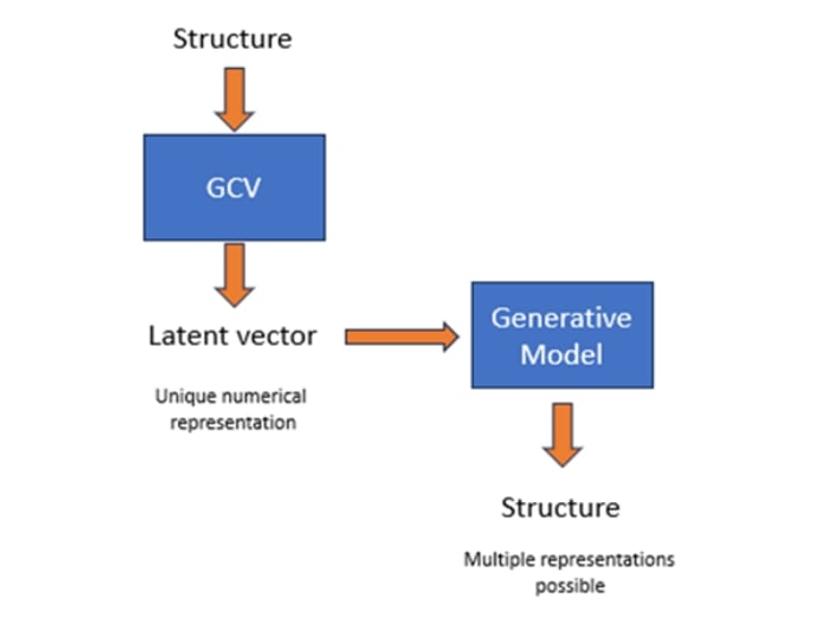
The model works by bridging the gap between two approaches to molecular representation, first converting a chemical structure into a numerical representation and then coupling this with a generative model able to produce simplified molecular-input line-entry system (SMILES) strings. “By coupling these two types of networks in one architecture, we can take the numerical representation resulting from a graph convolution vector and generate structures around it,” explains Gedeck.
This novel architecture supports several valuable applications, with similarity searching the first to become available in CDD Vault.
“Using the deep learning (DL) tool, we can create a numerical representation of a molecule, store it in a vector database, and then perform a similarity search against that,” adds Gedeck. “This is complementary to the more traditional similarity search methods, such as fingerprints and feature vector counts, but the search itself can be very fast – about a sub-second for several million structures.”
The DL tool has initially been integrated into CDD Vault for use with the ChEMBL dataset, allowing users to perform a similarity search against over 2.5 million synthesizable compounds, most of which have been tested against one or multiple drug targets. This can lead to insights about potential targets or side effect issues of novel screening hits.
According to Gedeck, one key advantage of having the DL tool integrated into CDD Vault is being able to securely conduct searches in public databases with proprietary structures. “All the searches are done in a trusted environment, so you can search in ChEMBL without exposing your structure information on a public server – which is a big win,” he says. “We are also considering incorporating vendor databases so that users have a way of expanding their structure-activity relationship (SAR) knowledge by ordering additional compounds.”
Beyond ChEMBL, the team is also in the process of expanding the integration to SureChEMBL, which will give users access to structures that are already patented, as well as links to the associated patents. “This is important because if researchers come across a hit in a high-throughput screening, they need to know whether this hit is covered by any other intellectual property (IP) or not,” Gedeck adds.
Using AI to tweak drug structures
In addition to extending the similarity search to a patent database and potentially vendor structures, CDD is also exploring the application of its deep learning algorithm to propose bioisosteric suggestions. Bioisoteres are molecules where one atom or group of atoms is substituted by another with similar properties, in a way that either conserves or enhances its biological activity.
“When we validated our system, we found that consistent structural changes – for example, changing a methyl group to a phenyl group – lead to similar changes in the latent vector space,” explains Gedeck. “We validated our latent vector representation also using a bioisostere database, and it works well, so we’re working to integrate it into CDD Vault as an idea generator.”
This will allow researchers to create several ideas around a structure by swapping out fragments with those that have a similar numerical representation.
“The advantage is it can create structures that are reasonable and not completely different from what you already have, whereas often the problem with generative models is they create something very different and that would be hard to synthesize,” Gedeck affirms.
Deep dive into SAR space to improve drug candidates
A final application of CDD's deep learning architecture, one that is still under development, is aimed at supporting the creation of quantitative structure-activity analysis (QSAR) models. Here, the numerical representations can be used to build DL models to predict the activity or chemical properties of molecules, helping to better understand how drug structure relates to drug-target interactions.

“We can also do something called inverse QSAR, where you have a numerical representation that, according to the QSAR model, should have better activity and you want to find the structure that represents it,” Gedeck enthuses. “By coupling the numerical representation with the generative model, we can create representation structures that then can be assessed.”
A two-dimensional representation of the SAR dataset can also be generated, with the results of the predictive model displayed with a heatmap. “The heatmap highlights unexplored areas, and by clicking on any spot, we can map this back to a latent vector and then generate a new structure for it,” he explains.
A new era of drug discovery
Artificial intelligence has the potential to revolutionize the process of drug discovery, signaling a new era in pharmaceutical research. Through CDD’s current and upcoming deep learning integrations within CDD Vault, biotech researchers can access the computational power to analyze vast datasets at an unprecedented speed, accurately predict molecular properties, and discover potential drug candidates using new and more cost-efficient methods.