Formulation workflows transformed with better data management
From spreadsheet silos to AI-powered insights, discover how Signals software is streamlining formulation science
2 May 2025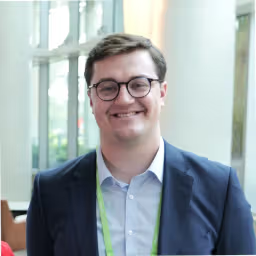
Across research and development, the way scientific data is generated, captured, and managed is evolving rapidly. As instrumentation becomes more advanced and experimentation more complex, traditional data handling methods are struggling to keep pace. According to David Gosalvez, Chief Strategy Officer at Revvity, the biggest barrier to innovation is not a lack of technology — it is how organizations manage their data.
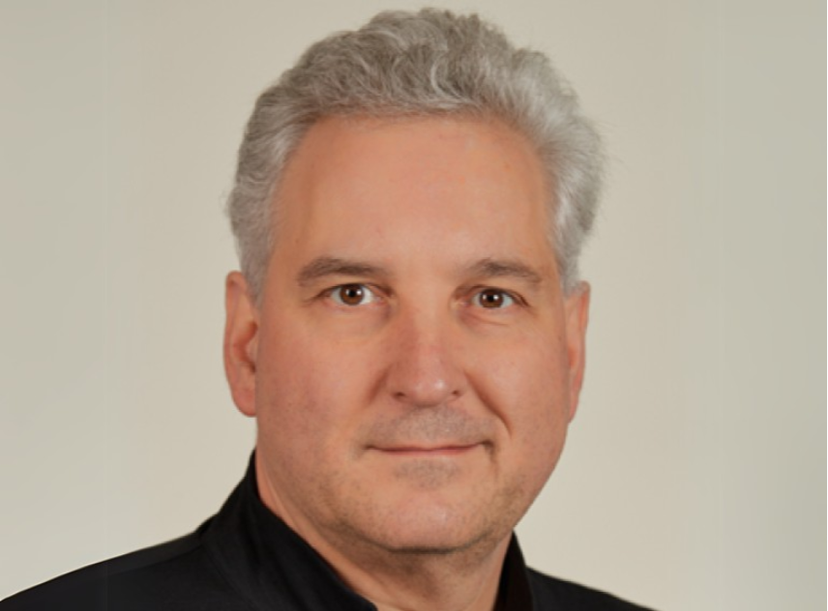
David Gosalvez, Chief Strategy Officer at Revvity
Challenges in formulation science
While data volume and complexity are increasing in R&D environments across all sectors, formulation science, especially in the food and chemical industries, faces distinct challenges. “In formulation science, it’s not so much about the volume of data, but about how well you capture and structure it,” Gosalvez explains. “The second challenge is how to reduce the number of physical experiments through prediction and in silico approaches.”
Formulation workflows are inherently iterative, often involving countless variations assessed in relation to critical quality attributes, cost, efficiency, regulatory compliance, and sustainability. The key to streamlining this, Gosalvez argues, is gaining efficiencies in that iteration process, which requires structured, accessible data from previous runs.
Want to excel? Leave it behind
Many organizations still rely on Microsoft Excel as the primary tool for managing formulation data. While flexible, Excel often leads scientists to record data in unstructured, inconsistent ways that make cross-comparison and trend analysis difficult. “When I walk into an organization and ask for their formulation data, they’ll typically hand over a bunch of inconsistently formatted Excel files. They might include some built-in calculations, but they’re usually siloed,” says Gosalvez. “You can analyze one Excel sheet, one experiment or one run but you're limited to a very narrow view, and there's huge value in extending it.”
In formulation science, this siloed approach drastically limits the ability to evaluate performance across experiments, let alone employ predictive modeling or AI to streamline formulation development. “Without a centralized system, it becomes impossible to analyze data across multiple experiments and select the best formulation from the ones you've actually run,” Gosalvez explains. “It doesn’t matter how good your models or algorithms are, if the data is in rough shape – unstructured, inconsistent, and siloed – you won’t get sufficient, high-quality data to run those models effectively.”
Enter, Signals Research Suite
Revitty’s cloud-based software platform – Signals One – was designed to directly address these challenges. Instead of relying on fragmented spreadsheets, Signals provides structured, purpose-built interfaces for capturing formulation data, calculating compositions, and storing everything in one centralized environment. This enables scientists to create, organize, and analyze formulation data more collaboratively and efficiently.
“One of the biggest benefits of that centralized and structured data is historical insight,” says Gosalvez. “You can learn from past experiments and avoid unnecessary repetition. That visibility is key for breaking down silos and unlocking the real power of analytics.”
Because Signals handles data capture, storage, and analysis in one environment, users no longer need to juggle multiple tools or reformat data for various analyses. Real-time dashboards provide insights during experimentation, while broader analytics evaluate performance across individual or multiple projects.
And for labs looking to take the next step – toward predictive modeling, machine learning, or AI – Signals lays the essential groundwork. “If you want to go beyond dashboards into machine learning, AI, or predictive modeling, having your data organized and accessible within Signals gives you the foundation to do that efficiently,” Gosalvez adds. “Without clean, accessible data, there's very little you can do with AI – no data, no value.”
Without clean, accessible data, there's very little you can do with AI – no data, no value.
Gosalvez Chief Strategy Officer at Revvity
Integrating specialized tools
Structuring and centralizing data is only the beginning. “What we’re seeing now, especially in the food industry, is a growing need for tools that can handle not just chemical formulation data, but also complex biological processes,” says Gosalvez. As food science moves beyond additives and preservatives into fermentation, microbiomes, and genetically influenced outcomes, the data itself becomes more complex – and so do the analytics required to extract meaning from it.
“There’s a lot more molecular biology in food R&D,” he adds, citing companies working with bacterial strains in dairy products or exploring sequence-level variations. These kinds of efforts require tools capable of handling large molecule data, bioreactor outputs, and sequence-based experimentation – capabilities that the Signals platform has developed over decades of supporting biotech and pharmaceutical R&D.
Here, Gosalvez highlights one of the key advantages of the Signals One as a SaaS (software as a service) platform: its ability to continuously evolve. “We can add capabilities to the software within weeks or months, and scientists are an integral part of the process,” he explains. “That’s why it’s so on point to what the scientists need, because they are the ones that are really influencing what we do.”
AI in action
Signals One is actively leveraging artificial intelligence to both transform how software functions and generate deeper scientific insight. “Large language models and generative AI have been rapidly embedded across the software, making it much more intelligent,” Gosalvez explains. These improvements might not always be flashy, but their impact is tangible: better search, quicker writing, and automation of previously manual, repetitive tasks. Whether it is auto-generating SOPs, summarizing data, or assisting with experimental prep, the AI is built into the scientist’s workflow. “It isn’t something they need to cut and paste into from a separate tool like ChatGPT,” Gosalvez adds.
Revvity is also working to integrate domain-specific algorithms into the platform. It embeds these tools where scientists work – whether inside ChemDraw ™, Spotfire®, or other domain-specific apps – removing the friction of exporting data and running models elsewhere. “Today, a lot of scientific AI is in the hands of computational scientists. We’re putting it in front of the bench scientists too,” Gosalvez says. “And we’re not trying to build all of these models ourselves – we’re trying to act as a conduit. The best algorithms I’ve seen are done by computational scientists with deep expertise in their specific area.”
Future outlooks
Looking ahead to the impact of Signals, Gosalvez sees a clear opportunity to help formulation scientists run better, more efficient experiments. With greater coverage and structured experimentation, researchers are better equipped to identify the right formulations with confidence.
He also points to the power of predictive analytics to reduce unnecessary wet work, freeing up time, resources, and budget. “Reducing the wet work they do – where they spend the most money – is going to give the fastest impact,” he explains.
Most importantly, he underscores the value of continuous improvements. Whether it is a new model, a custom calculation, or a simple interface tweak, the goal is to shape the platform around scientists’ real-world needs. “One scientist at a time,” he says. “That’s how we make sure the platform continues to be the best for the scientists themselves.”