How to train AI for drug development
In this guest editorial, we learn how to develop an AI's mindset to facilitate drug development from an idea to patient, with BMX target as an example
5 Oct 2023
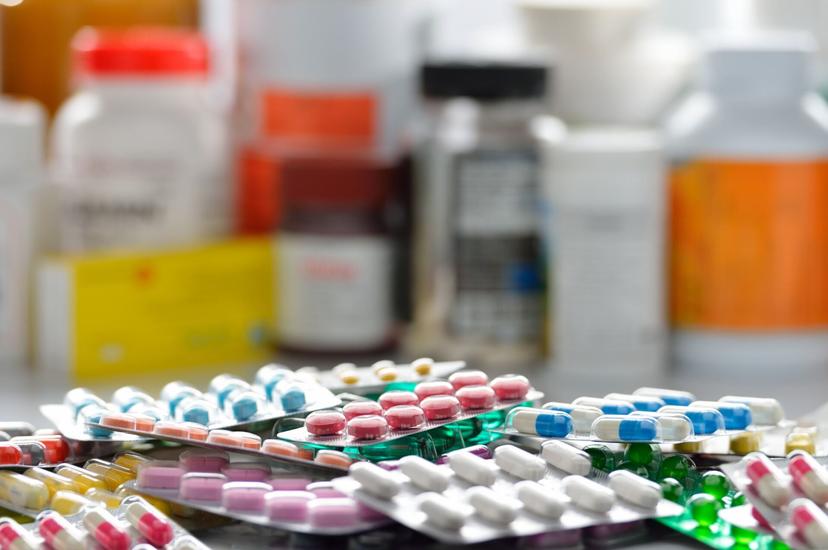
Today, we live in the era of data. Such data is important in all aspects when it comes to product developers. While the huge availability of data might facilitate the development process, mining tools and algorithms are extremely important to streamline such efforts. The simulation of human intelligence by soft- or hard-wares, which is currently known as artificial intelligence (AI), has tremendously accelerated the results-oriented mining of such data.
In health care, the pharmaceutical product development process is a lengthy and extremely costly initiative. Interestingly, even in today's numbers, it costs almost $1 billion and at minimum 10 years to get a molecule from discovery to FDA approval. It is true that worldwide efforts are being implemented to maximize leverage on the current huge-data availability and using AI to facilitate the drug development process. For example, several new biotech startup companies have recently founded pipelines that are primarily based on AI in discovery and development. Moreover, it might be beneficial to the scientific and product developers’ community to share an example on that implementation from an academic lab pursuing a discovery and translation industry-tailored program employing AI in the process.
It is also worth noting that academia in general has limited funding capability. Recently, there has been a preliminary IND-enabling study of selective BMX inhibitors for cancer therapeutics development1. BMX is an emerging target, more notably in oncological and immunological diseases. In this work, a predictive AI-based platform has been employed to design the selective inhibitors considering the novelty, IP prior protection, and drug-likeness properties. Using the AI-based platform, several candidate molecules that are selective to the target of interest were discovered. Interestingly, some of the top active molecules were synthesized, and experimentally tested using enzymatic assays and cancer cell lines, and data were amazingly matching the AI-initially collected data.
The top selected molecules were further AI-tested using several freely available online platforms to predict IND-enabling level parameters, including CMC-physicochemical properties, oral bioavailability, safety pharmacology and toxicity profile prediction. All predicted IND-enabling data amazingly showed favored drug-like characteristics that encourage the further move forward in development. Such AI-collected data were also compared to already FDA-approved products currently on the market. Moreover, all these IND-enabling data are currently being verified in real experimental procedures.
An AI-based platform to predict the clinical trial-related enrolment of a future clinical study is currently being piloted to evaluate such molecules in patients who are precisely in need of such therapeutics. Such AI-based clinical moves add more added value to patients’ care and drug development process. It is what is currently known as personalized data. AI can further advance personalized medicine, and diseases to be more precisely treated. Data regarding employing AI in such clinical moves will be further communicated with the scientific and drug developers’ community as it becomes available.
In summary, data science and AI have undoubtedly great futures, and more champions are extremely in need to step in to test and validate such amazing approaches. AI will certainly open the doors for all product developers in all disciplines towards new ways to accelerate the development and launch new products, where humanity gets the benefits of faster.
This guest editorial article was written by Dr. Ahmed Abu Fayyad, an Associate Professor of Pharmaceutics at Long Island University.
References:
Elsanhoury R., Alasmari A., Parupathi P., Jumaa M., Al-Fayoumi S., Kumar A., Khashan R., Nazzal S., Fayyad A.A.. AI & experimental-based discovery and preclinical IND-enabling studies of selective BMX inhibitors for development of cancer therapeutics. International Journal of Pharmaceutics. 2023 Oct 15;645:123384.