King’s College London accelerates synthetic brain 3D image creation
The Synthetic Brain Project is using AI models powered by the Cambridge-1 supercomputer
29 Jul 2021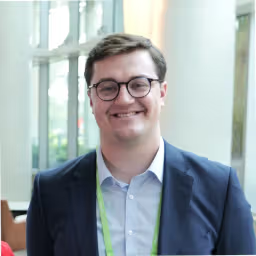
King’s College London, along with partner hospitals and university collaborators, has unveiled new details about one of the first projects on Cambridge-1, the United Kingdom’s most powerful supercomputer.
The Synthetic Brain Project is focused on building deep learning models that can synthesize artificial 3D MRI images of human brains. These models can help scientists understand what a human brain looks like across a variety of ages, genders, and diseases. The AI models were developed by King’s College London and NVIDIA data scientists and engineers as part of The London Medical Imaging & AI Centre for Value-Based Healthcare research funded by UK Research and Innovation and a Wellcome Flagship Programme (in collaboration with University College London).
The aim of developing the AI models is to help diagnose neurological diseases based on brain MRI scans, but it may also be used to predict diseases that a brain may develop over time and enable preventative treatment. The use of synthetic data has the additional benefit that it can ensure patient privacy since the images were generated and it will allow King’s to open the research to the broader UK healthcare community. Without Cambridge-1, the AI models would have taken months versus weeks to train and the resulting image quality would not have been as clear. King’s and NVIDIA researchers used Cambridge-1 to scale the models to the necessary size using multiple GPUs, and then applied a process known as hyperparameter tuning, which dramatically improved the accuracy of the models.
“Cambridge-1 enables accelerated generation of synthetic data that gives researchers at King’s the ability to understand how different factors affect the brain, anatomy, and pathology,” said Jorge Cardoso, Senior Lecturer in Artificial Medical Intelligence at King’s College London. “We can ask our models to generate an almost infinite amount of data, with prescribed ages and diseases; with this, we can start tackling problems such as how diseases affect the brain and when abnormalities might exist.”
The introduction of NVIDIA’s Cambridge-1 supercomputer poses new possibilities for ground-breaking research like the Synthetic Brain Project. King’s College London and other leading healthcare institutions will use Cambridge-1 to accelerate ground-breaking research in digital biology on disease, drug design, and the human genome.
Cambridge-1 is one of the world’s top 50 fastest supercomputers, built on 80 DGX A100 systems, integrating NVIDIA A100 GPUs, Bluefield-2 DPUs, and NVIDIA HDR InfiniBand networking.
King’s College London is leveraging NVIDIA hardware and the open-source MONAI software framework supported by Pytorch and NVIDIA’s software solutions like cuDNN and Omniverse for their Synthetic Brain Project. MONAI is a freely available, community-supported, PyTorch-based framework for deep learning in healthcare imaging, NVIDIA CUDA® Deep Neural Network library (cuDNN) is a GPU-accelerated library for deep neural networks, and NVIDIA Omniverse™ is an open platform for virtual collaboration and real-time accurate simulation. King’s has just begun using Omniverse to beautifully visualize brains, which can help physicians better understand the morphology and pathology of brain diseases.
The increasing efficiency of deep learning architectures, together with hardware improvements, have enabled the complex and high-dimensional modeling of medical volumetric data at higher resolutions. Vector-Quantized Variational Autoencoders (VQ-VAE) have been an option for an efficient generative unsupervised learning approach that can encode images to a substantially compressed representation compared to its initial size, while preserving the decoded fidelity. King’s used a VQ-VAE inspired and 3D optimized network to efficiently encode a full-resolution brain volume, compressing the data to less than 1% of the original size while maintaining image fidelity, and significantly outperforming the previous State-of-the-Art (SOTA).
After the images are encoded via the VQ-VAE, the latent space is learned via a long-range transformer model optimized for the volumetric nature of the data and associated sequence length. The sequence length caused by the 3-dimensional nature of the data required unparalleled model sizes that were only made possible by the multi-GPU and multi-node scaling provided by Cambridge-1. By sampling from these large transformer models, and conditioning on clinical variables of interest (age, disease), new latent space sequences can be generated, which can then be decoded into volumetric brain images using the VQ-VAE. Transformer AI models adopt the mechanism of attention, differentially weighing the significance of each part of the input data, and were used because they understand these sequence lengths well. Creating these generative brain images that are eerily similar to real-life neurological radiology studies (examples here) can be ground-breaking when understanding how the brain forms, how trauma and disease affect it, and how to help it recover. Instead of real patient data, the use of synthetic data can also help mitigate problems with data access and patient privacy.
As part of this synthetic brain generation project, King’s College London will make the code and models available as open-source and NVIDIA has made open-source contributions to improve the performance of the fast-transformers project, on which The Synthetic Brain Project depends on.
Want the latest science news straight to your inbox? Become a SelectScience member for free today>>