Leveraging data analytics to derive valuable insights
Watch this on-demand webinar to discover the benefits of implementing new data analytics, visualization tools and techniques in the lab
4 Mar 2022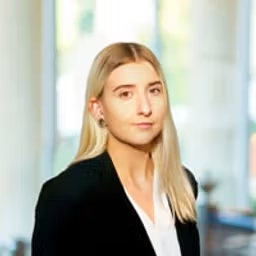
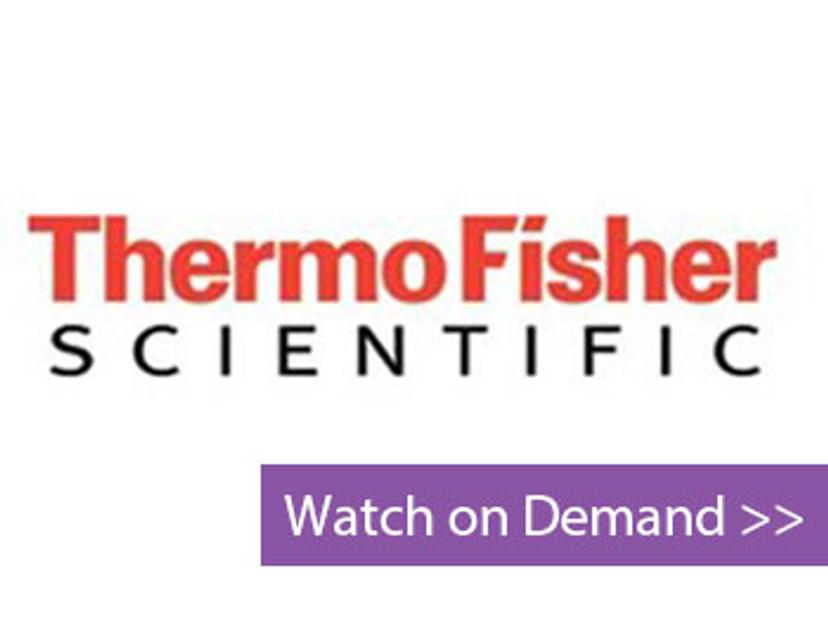
Effective data analysis is critical to provide scientists with actionable information and valuable insights. Although organizations are utilizing data analytics tools from a business intelligence perspective, there is the opportunity to leverage data even more and use it to drive decisions.
Unfortunately, a lot of data is not utilized fully because it does not follow the FAIR (findable, accessible, interoperable and reusable) guiding principles for scientific data management and stewardship, making it unusable. Open standards from Allotrope, the Pistoia Alliance and the American Society for Testing and Materials (ASTM) all help to “FAIRify” data, making it available for wider use.
Once the power of data is unlocked, laboratories can begin to experience the full value of technological advancements, including predictive analytics, artificial intelligence, and machine learning tools.
In this on-demand SelectScience® webinar, Dr. David Hardy, Thermo Fisher Scientific, reveals the value of data analytics and visualization tools and explains how laboratories can effectively use these to optimize their data to drive efficiency and innovation. Plus, Dario Rodriquez, from the data analytics and visualization team of the Analytical Instruments Group, shares his experience as a data scientist in the Q&A session. This webinar is the third of our 10-part webinar series, The Orchestrated Lab, being run in partnership with Thermo Fisher Scientific.
Watch on demandRead on for the live Q&A session or register to watch the webinar at a time that suits you.
Can you expand on explainability of machine learning tools?
DR: In machine learning, there's a tradeoff between explainability and accuracy of the models. Meaning the higher the accuracy, the more complex the model is. We can think about networks with lots of parameters and when you have these complex models, it ends up being like a black box where you can see the inputs and outputs.
On the other hand, we've got simpler models like linear or logistic regressions, or even a decision tree, that give you a more understandable result, making it easier to understand how the model works. You can also have more insights into the variables that play in their predictions. When you're deciding on which machine learning tool to use you should always have in mind if you're aiming for more explainability process, in order to understand what's going on, or if you're focusing on the predictive side of the models.
We run several tests to assess the quality of our products. How are you able to reduce the number of tests and continue assessing the quality?
DR: We start with the premise that the quality, or whatever characteristic you're measuring, is a combination of different properties that interact with each other. You’ll do a lot of tests to assess the quality and each of these tests has a predictive power by itself and in combination with each other.
The idea is to start with the test with the highest individual predictive power, and then predict the quality. If that prediction is lower than what you're expecting, you could stop right there and say, ‘Okay, the sample doesn't get that high.’ For example, we would reject it. But if it’s not that low, we can continue with the next test that gives the best predictive power and so on.
Some samples may need more resources than others, but you may encounter samples where you only need to do one or two tests, so you save a lot of testing time. This is just focused on predictive power, but you should combine the cuts of the test with the predictive power to get the optimal process for quality assessment.
How accurate are the sample predictions, for example? And how do you know they can be used in different environments?
DR: When implementing a machine learning solution, the best practice is to train body data. And finally, test its results with data that has been unseen by the model, and has similarities or the same distribution with the data you're expecting to receive in the future.
When you do this testing of your algorithm, you can assess the performance of the machine learning model. In some cases, the algorithm may not perform well in different environments or locations due to multiple reasons. There may be noise or missing values in some specific locations or labs, for example. We always make sure that each algorithm is tested in each environment. Therefore, you will have the specific metrics for the place you're interested in. Then you can decide if that metric is okay for you, depending on the problem you want to solve.
What are the precautions to take regarding the GDPR?
DH: There’s this idea that the data is just thrown into a data lake and there's no security, when there is. All around the data lake we have permissions and security.
What we've tried to ensure is that yes, we're storing data in a data lake in a standardized format, but we're still using tools that enable that security and governance. For example, the permissions and roles, etc., are still applied equally across the data lake as it would be in the SampleManager LIMs database, for example.
Do you think all vendors will get around to producing a common data format?
DH: I think that vendors are ensuring that all the data is available in a single format, probably as an export. For the large companies, our customers are pushing us for that. The data has different nuances due to hardware, for example, the way they calculate signal to noise. I don't think we'll ever all be producing a single, unified data format, but we can certainly convert it.
Learn more about The Orchestrated Lab series and register your place for upcoming webinars.
Find out more about how to leverage data analytics to benefit your lab, watch the webinar on demand >>