Spotlight on the impact of artificial intelligence on drug discovery
From meeting challenges in solution prep to more 'flashy' analysis of the interaction between drugs, humans, and their contexts, we learn about the mounting importance of AI in drug discovery
30 Sept 2019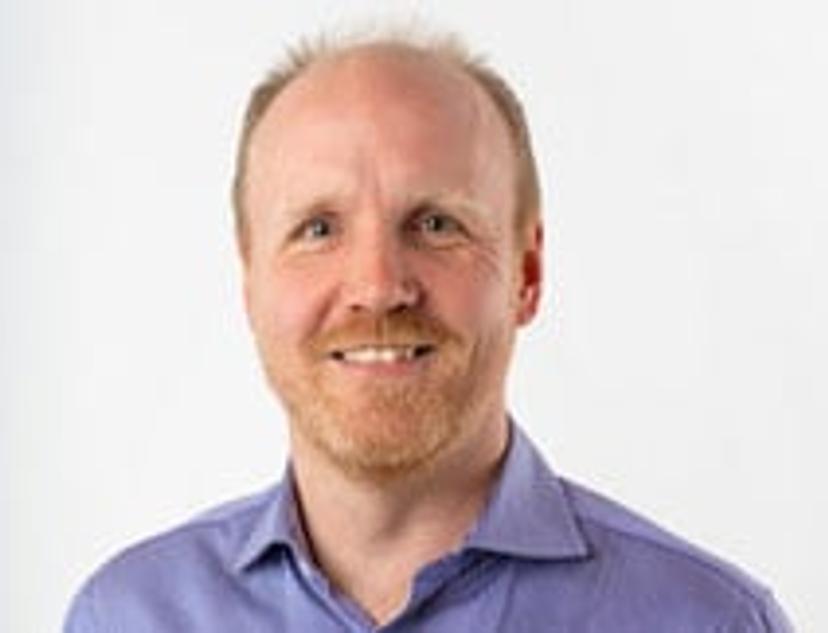
With the use of artificial intelligence becoming ever more prevalent across multiple industries, what can we look forward to from AI within the realms of drug discovery? From Nobel Prizes to high-profile analysis of results in real populations, there's an exciting future on the cards, according to Ville Lehtonen, founder and CEO of LabMinds. But it won't all be illustrious awards and frontpage headlines, there will be some hugely practical solutions for scientists in the field too, resulting in faster development cycles and more efficient lab processes. Here, ahead of his Lightning session at the SLAS 2019 AI in Process Automation Symposium in Boston, USA, Lehtonen talks about LabMinds' developments in AI technology, the need for collaboration and what the future holds.
What are the current challenges in solution preparation in drug discovery?
VL: At the drug discovery stage, when drug candidates are screened against biological targets, solution replication is essential to ensure successful experiments and guarantee trusted results. In order to do this, strategies should be developed to get ahead of some major challenges. Quality of chemical input is one of the first challenges. Some chemicals end up catching meaningful amounts of unintended water in them, resulting in molarities that can be 10% off what's expected, which you have to be aware of.
Secondly, from a sensor perspective, particularly with pH sensors, there can be a significant error stack. This explains why a lot of old recipes do not work as intended; it’s highly likely there was something going on with the pH probe when the recipe was being developed, so there is very little statistical ruggedness. (Ideally, you would run the solution five to ten times on three different pH probes, each calibrated with different pH standards).
Thirdly, at LabMinds, we focus on the output values, rather than the molarities and percentages. This is because when it comes to individual solutions, balances, and visual inspection, focusing on molarities and percentages feels more intuitive, leaving more room for error.
And finally, formulation can be remarkably slow, especially if you want your results to be robust enough to establish solid recipe maps for the whole space. Automation not only eliminates the manual operation but also improves accuracy and throughput. We propose that if you know what you're targeting, the AI could go straight to those properties without having to test around, vastly simplifying your life and speeding everything up.
Tell us about the unique features of the LabMinds Solution Recipe Platform (SolReP). How does it address the challenges in solution preparation? How does AI benefit the user? Which applications and industries will the SolReP benefit most?
VL: LabMinds’ Solution Recipe Platform is the brain inside Revo that allows our end-users the ability to order their solution at targeted pH, which our AI will achieve on a consistent basis. One of the most obvious ways that AI benefits the users of our system is that Revo recognizes and reports any meaningful statistical variations from the noise of the various sensors. Input and sensor anomalies are quite cumbersome to track, so in the cases where there are issues, they are easy to miss. With Revo, when there is a problem, self-diagnostics can be triggered, creating a self-validating process.
If you are looking to try a lot of combinations and would like to get a better understanding for how to reach certain results, Revo is the technology you are looking for. Its ability to catch anomalies based on sophisticated statistical analysis is extremely high value and would serve any laboratory, from Formulations to QA/QC groups, regardless of industry.
Tell us a little about your upcoming lightning session talk at the SLAS 2019 AI in Process Automation Symposium? Who should come to the session and how will they benefit?
VL: We’re going to touch on some of the issues LabMinds initially aimed to tackle with Revo, plus a couple of problems we uncovered in the merciless light of AI analytics. And of course, we’ll discuss how we solved them.
LabMinds does AI industry-wide, rather than in organization-wide silos. This has some interesting challenges, but is obviously the right way to solve problems due to the far higher amounts of data. If anyone wants to learn more about the intersection of chemistry and huge amounts of data, they should come to this session.
What are you looking forward to most about the AI in Process Automation Symposium?
VL: I am really interested in seeing how AI is done elsewhere, and I look forward to seeing if there are opportunities for LabMinds to collaborate. This is a rather challenging prospect from a technical perspective, but it wouldn’t hurt to start the conversations.
The thing is, LabMinds is in a unique position: our system is not a rival, or really in parallel, to anyone else; we are positioned upstream from the rest. This means that data flow could ultimately lead to self-iterating systems with an automated physical component tied into it. Essentially, labs could conduct physical experiments without humans present, paving the way to an actual automated laboratory.
How do you see advances in AI impacting the drug discovery industry in future?
VL: First, it’s important to note that there are different types of AIs being used.
The ones like LabMinds' AIs will become ubiquitous quite quickly, and their impact will be subtle; we’ll see it show up in failure rates, which should result in faster development cycles. This sounds boring, but the impact might well be savings in the range of 50%-80% for all the AIs combined. Actual drug development AIs will also have a similarly quiet effect, mainly allowing people to focus on doing slow, physical research on the right thing.
These two will behave a little like Moore's law. The efficiency of lab processes will keep going through the roof, but it won't be obvious why, because a lab in 2025 being guided by the best AIs available won't be in any way different from an extremely lucky lab in 2015. Except, of course, only a small percentage of labs are that lucky, while every lab can use the AIs. The way these two will get public attention is when Nobel prizes are won, which I predict won’t actually take that long. I would be disappointed if, by 2030 at least, one hasn't been given for an AI development in these spaces.
The most interesting AIs from a journalistic and human-centric perspective will be the ones analyzing results in real populations and figuring out the interaction between drugs, humans, and their contexts. The results from these will be very flashy and will benefit enormously from being very easy for the general public to understand, unlike the rather more dry results of the previous two groups. The insights from these will be the big drivers and probably will attract a lot more of the generalist funding due to it.
If you're attending SLAS 2019 AI in Process Automation Symposium be sure to join Ville Lehtonen for the Lightning Session- Employing Supervised Learning Methods To Optimize pH Targeting In Chemical Solutions at 2.20pm on Thursday October 3, 2019.
Register for your free SelectScience membership today to receive the latest editorial articles and technology news direct to your inbox>>