Enhancing biomarker analysis with bioinformatics: lessons in an emerging field
The head of bioinformatics at Sengenics reveals more about the emerging field of bioinformatics, and how the knowledge gained can be applied to enhance immunoprofiling to find meaningful biomarker signatures
4 Jan 2023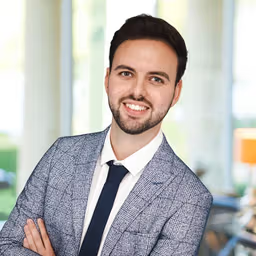
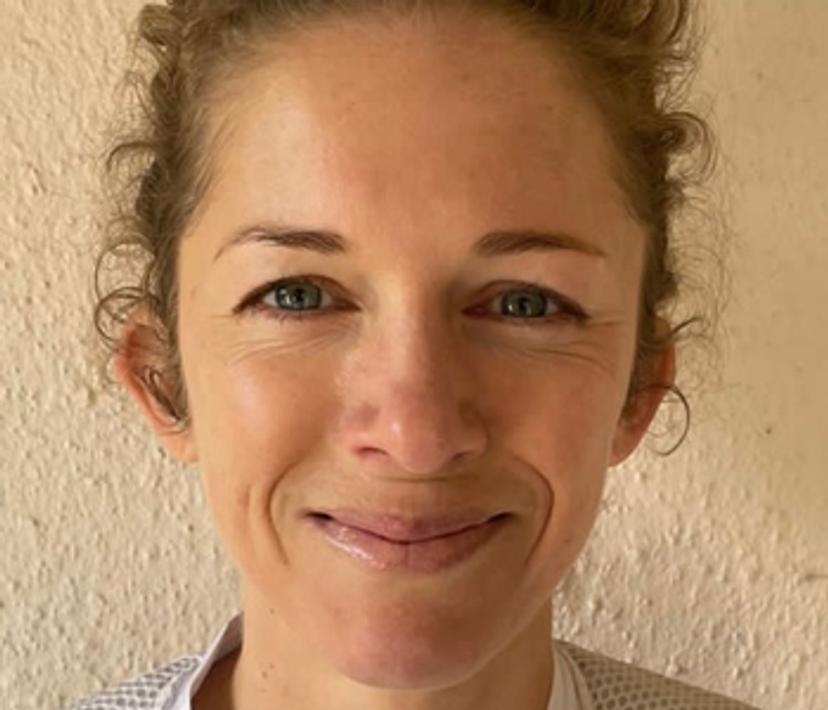
In this exclusive SelectScience® interview, we speak with Dr. Katie Lennard, head of bioinformatics at Sengenics, to find out more about the evolving field of bioinformatics, which uses complex software to retrieve, analyze, and store biological data. Lennard will also explore how this scientific subdiscipline has proved valuable to improving autoantibody biomarker discovery and reveal more about her role as a bioinformatician. Plus, Lennard will explain how the talented team at Sengenics have been working hard to streamline their data analysis pipeline, in order to analyze client data and generate high-quality results that are both easy to interpret and clinically meaningful.
The emerging field of bioinformatics
Bioinformatics is an exciting and evolving scientific subdiscipline, which involves the application of software and computational approaches to collect, store, and analyze large quantities of biological data and information. This discipline is now intrinsic to most areas of scientific research, allowing us to make sense of large, complex data sets like never before. Interestingly, this field encompasses a whole range of other scientific disciplines, from biology and medicine to mathematics and computer science. Bioinformatics relies on the use of several analytical tools for both data preprocessing (QC, data filtering and normalization) and downstream analyses (e.g., biomarker panel identification and between-class comparisons).
We brainstorm on a regular basis to come up with new ideas to streamline our data analysis pipeline and analyze client data, so that we can produce high-quality results, that are easy to interpret, and clinically meaningful.
Dr. Katie Lennard
Sengenics
Helping to further advance this field is Dr. Katie Lennard, who has already gained a wealth of experience in this emerging area. “I earned my PhD in medical biochemistry at the University of Cape Town, where I focused on identifying microbes associated with transcriptional changes in colorectal cancer. Out of necessity, I had to analyze my own whole genome transcriptomic and genome-wide methylation data. This is when I started acquiring bioinformatics skills through short courses and self-learning,” explains Lennard. “I quickly realized that my interests were better suited to data analysis and bioinformatics, compared to wet lab work.”
Lennard has been fascinated with bioinformatics for many years and has always been drawn to big data. “I love problem-solving and finding meaningful patterns in complex data and developing bioinformatics solutions suited to specific research goals. I think my interest in bioinformatics was a natural evolution from my initial start as a wet lab scientist.”
At Sengenics, Lennard leads a talented team of bioinformaticians with diverse skill sets. “We work together really well, brainstorming on a regular basis to come up with new ideas to streamline our data analysis pipeline and analyze client data, so that we can produce high-quality results, that are easy to interpret, and clinically meaningful,” she says.
Bioinformatics in personalized medicine
A biomarker is a biological characteristic that can be objectively measured and used as an indicator of disease, to stratify patient subgroups, or to predict response to treatment. Identifying biomarker signatures can help clinicians better understand cancers and other diseases to improve patient management and outcomes. Bioinformatics is essential for the analysis of multi-omics biomarker data– this approach now serves as a significant step toward the goal of personalized medicine. “Bioinformatics provides us with the appropriate analytical tools to identify clinically relevant biomarkers in complex datasets. This relates to initial data quality control and processing, as well as implementing the eventual tools, like machine learning, that we will use to select biomarkers,” explains Lennard.
Bioinformatics provides us with the appropriate analytical tools to identify clinically relevant biomarkers in complex datasets.
Dr. Katie Lennard
Sengenics
There are many different types of molecules that serve as biomarkers, these can be contained within a range of biological fluids, from serum and plasma to saliva and urine. Therefore, this means that the approach used to analyze different biomarkers can differ. “Broadly speaking, we use the same approach for, let's say, proteomics, transcriptomics, or autoantibody data. However, the customized approaches to preprocessing the data, and to the pre-filtering features, are dictated by the specific structure of the data, which differs greatly between something like Sengenics’s immunoproteomics data vs. something like transcriptomics data. This is where the customization really comes in,” states Lennard.
Immunoproteomic biomarker profiling (based on protein microarray-antibody binding assays) requires a more specialized approach. Autoantibodies are antibodies that target self-proteins. This may occur for various reasons, including over-expression of a protein, aberrant protein variants (e.g. cancer) or molecular mimicry. Autoantibodies may or may not be associated with pathology and some are even protective! “Think of your antibody repertoire (which includes autoantibodies) as a mix of germline encoded antibodies and those that were elicited in response to a foreign antigen or neoantigen. This presents us with a unique opportunity to build a proteomics-based immune profile, which is not possible through, for example, gene expression data or mass spectrometry-based proteomics. Broadly speaking, our technology can be used for functional profiling (improved understanding of potential disease etiology and/or progression) and clinical applications (predictive, diagnostic biomarker candidates and identifying potential pathogenic or protective antibodies).
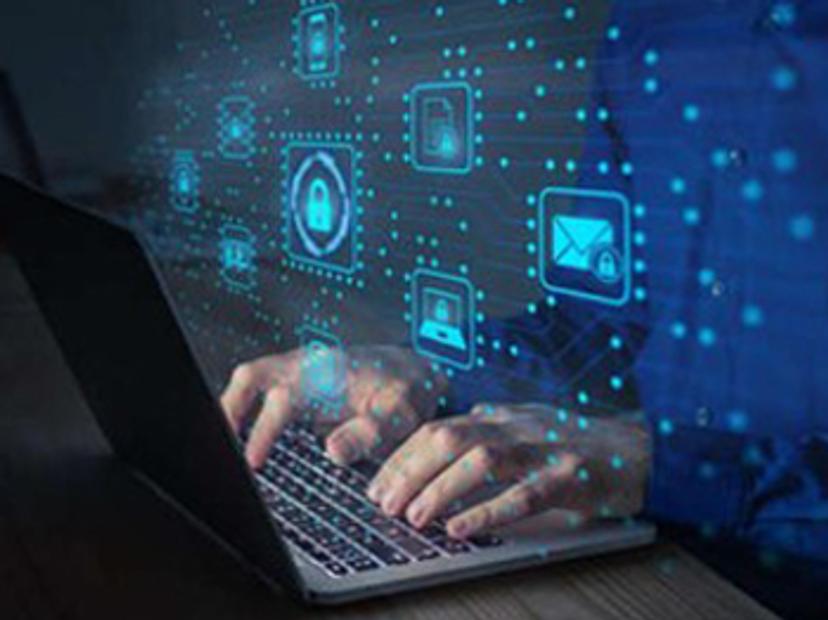
“In terms of bioinformatics, given that autoantibody profiles are unique to an individual, the resulting data presents very differently to, for example, transcriptomic data, where we see much more consistent signals or expression levels across individuals within a given class of interest,” explains Lennard. “So, when we're comparing classes of interest, significant hits often present as consistent blocks on a heat map. This is not the case for autoantibody data, which makes the downstream analysis slightly trickier. In this case, you can think of the data as consisting of two layers. So, the first layer would be low-level noise, intrinsic to any microarray experiment, which may be further influenced by technical factors, like slide batch; whereas the second layer would be the true autoantibody data, which is what we're interested in. The latter presents as a salt-and-pepper type of pattern when visualized as a heat map.” Therefore, the distribution of data can appear very different to gene expression data. For this reason, Lennard and her team at Sengenics have developed a custom pipeline to identify true autoantibodies. “The selection of these features occurs independently of sample information, or any form of normalization – which ensures an objective selection of biologically relevant features that we'll use for downstream machine-learning based biomarker discovery,” she says.
Looking ahead
The Sengenics bioinformatics team is currently focused on developing a user-friendly interface for data preprocessing, downstream analyses, and interpretation, that will be useful for anyone that wants to analyze Sengenics data themselves. “A large part of our time goes into delivering client results, so this includes multivariate analysis, machine-learning, biomarker panels, and exploring disease subtypes by unsupervised clustering methods. As part of our analyses, we also come up with new ideas for future products based on the data that we see,” explains Lennard. In terms of whether bioinformatics informs product development, Lennard concludes by saying, “We've found through experience that a well thought-through bioinformatics analysis platform often brings to light certain details that either inspire new product ideas or can be used to further refine our assays in the lab.”