Using DNA repair and machine learning to improve cancer risk prediction
Scientists are harnessing machine learning to make accurate measurements of breast cancer risk from a patient’s blood cells
5 Jul 2020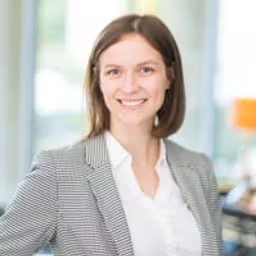
Across the world, breast cancer continues to be the leading type of cancer in women, accounting for 25% of all cases. In the fight to stop breast cancer from taking lives, research organizations and charities are investing increasing funds into better understanding cancer risk and then using that information to prevent the disease from gaining a foothold. Current predictors of risk, such as mutations in specific genes, can only take us so far, and a step change is needed to both raise that prediction factor and produce the technology to measure it accurately from biological samples.
A laboratory in the US has designed a DNA repair assay that might do just that. Working out of the Center for Radiological Research, Columbia University Irving Medical Center (CUIMC) in New York, Dr. Bezalel Bacon is a postdoctoral biochemist analyzing blood cells from people who donated blood 20 years ago to see how predictive their DNA repair activity may be of future health or disease. But his studies are ‘blind’. Only his colleagues in the epidemiology department know the provenance of the samples and the health or disease pathway traveled by the donor in the intervening years. Allied to this rigor is the innovative application of machine learning to cutting-edge imaging flow cytometry, which is proving to be a ‘game-changer’ in cancer prediction.
Predicting breast cancer risk
Bacon explains that the classical approaches to understanding susceptibility to breast cancer, such as family history and specific mutations in genes such as BRCA and HER2, are only 60-70% reliable for predicting risk. And given the unpleasant side effects that some cancer treatments can have, decisions to start treatment without the evidence of any real cancer symptoms are not taken lightly. However, improving that predictivity through DNA repair analysis changes the equation significantly. “The projection from the epidemiology side was that this would be a greater-than-83% prediction factor,” Bacon says.
Analyzing DNA repair in thawed-out blood cells from samples donated 20 years ago, however, was never going to be easy. “We’ve optimized this method for thawing the cells that enables them to survive for the experiment — the first tricky part of the project,” says Bacon. The next step was no less challenging, working out just how much to irradiate the cells to cause enough DNA damage to see an effect without rendering the cells unable to repair themselves. Once that ‘sweet spot’ was determined, as Bacon calls it, “the cells of healthy people should be able to repair the amount of damage we’re causing,” he explains. The key to predictivity of future cancer risk then lies in measuring the DNA damage and how quickly it is repaired. And that relies upon Bacon’s use of Luminex’s pioneering imaging flow cytometer, the Amnis® ImageStream®X Mk II, allied to groundbreaking machine learning in the Amnis IDEAS 6.3 image analysis software.
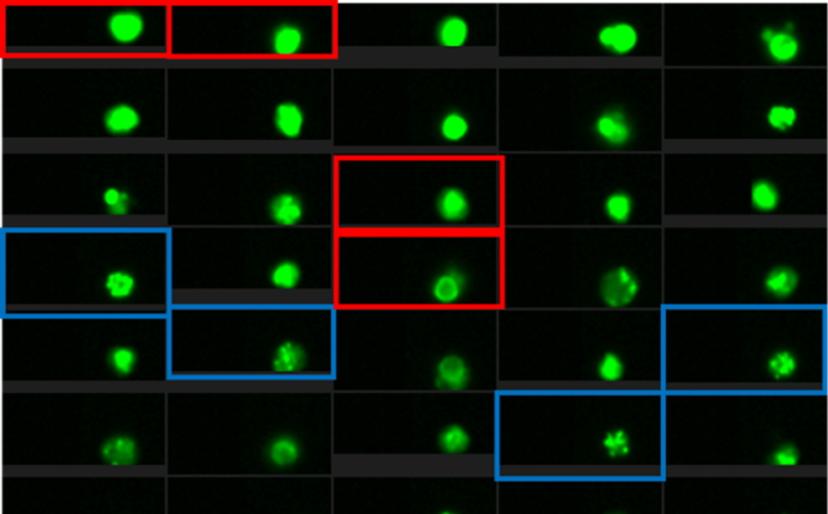
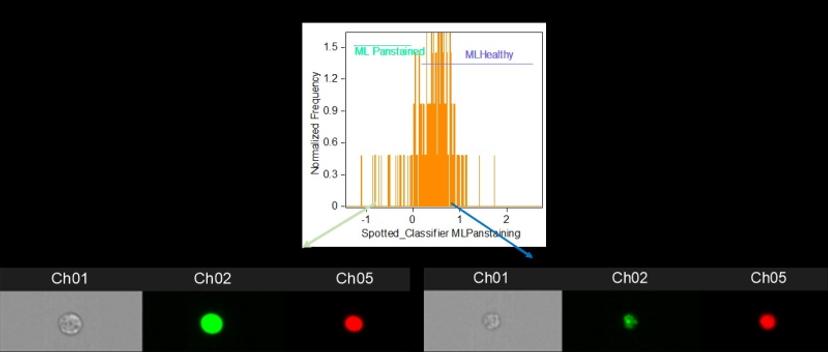
The impact of machine learning
The project uses a protein marker recruited to the site of DNA damage and bound with a fluorescent antibody to analyze DNA repair over time following irradiation. The decay, or not, in the fluorescence signal is indicative of the efficiency of normal DNA repair by the cells. Traditionally, this might have been achieved using microscopy, which entails slow signal analysis and a limited number of cells that can be analyzed, or by flow cytometry, which is fast and simple, but lacks the nuanced detail of microscopy. Bacon alludes to his “game-changer for the whole project” as he describes Luminex’s latest technology: “Luminex combines microscopy and flow cytometry with a new technology where you get the microscope information for every cell and then the software is able to actually analyze that much data.”
Luminex combines microscopy and flow cytometry with a new technology where you get the microscopic information for every cell and then the software is able to analyze that data
Bacon is referring to the addition of a machine learning module to the Luminex Amnis® ImageStream® X Mk II which, he says, “provides a lot more precision and subtlety in how you’re selecting out your cells.” And streamlining and precision are vitally important, as Bacon explains how the very small difference in fluorescence signal between a healthy person and a less healthy person often comes down to the 24-hour time point after irradiation, when most healthy cells should repair their DNA. As a result, Bacon is in no doubt about the benefits of Luminex’s machine learning module, asserting that “it’s making something possible that I don’t think is really possible without the machine learning.”
Another advantage of working with Luminex has been the knowledgeable support on offer. “You’re speaking to scientists who are able to understand your experiment, what your goal is, and they’re able to walk you through some of the nuances of the software you might not have realized are possible,” says Bacon. “They can also walk you through fixing some problems on your own, so I’d say that Luminex support has been very good.”
Pulling all the DNA repair time points together enables Bacon to generate kinetic parameters for each cell donor which are passed on to the epidemiologists for an improved prediction of breast cancer risk.
Future possibilities
Bacon concludes with an exciting prospect for the use of machine learning allied to cutting-edge imaging flow cytometry. “It’s opening up avenues of research that were not previously plausible,” he says.
He describes the “vast sea of information” that emerges from the Luminex Amnis® ImageStream® X Mk II and how machine learning provides the means of navigating that sea. New knowledge is likely to abound, as Bacon asserts: “Just in this one project, I could write four different papers on the data which has come out from it.”
Do you use Luminex products in your lab? Write a review today for your chance to win a $400 Amazon gift card>>