Integrating artificial intelligence with digital laboratory tools to revolutionize life sciences
Discover how digital laboratory tools provider eLabNext is teaming up with artificial intelligence (AI) software companies to create new, integrated AI laboratory solutions
14 Jun 2023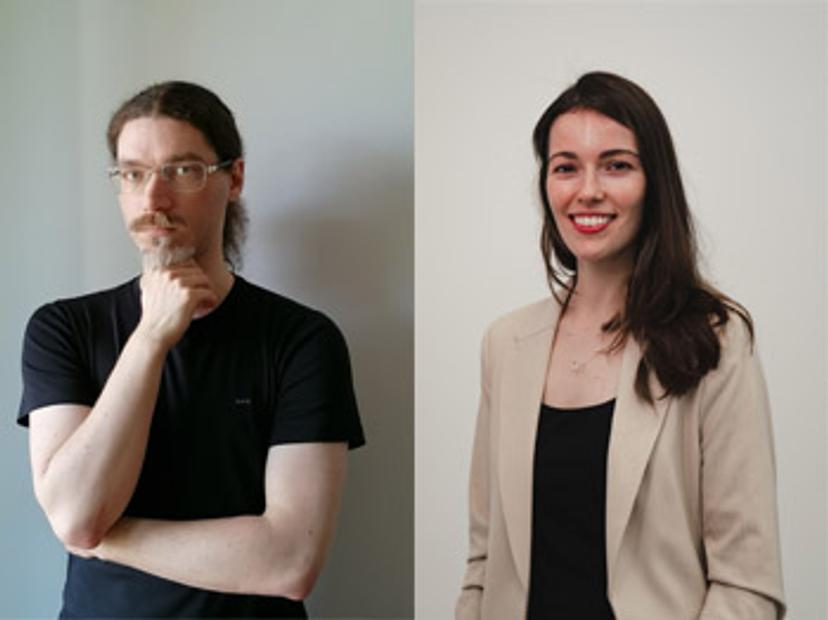
A revolution is underway in the life science and biotechnology industries, harnessing AI to increase laboratory efficiency, speed up process development workflows, and ultimately, enable discoveries in cancer research, vaccine development, and much more. However, research and development companies are not internally equipped to build and scale AI software, so the necessary solutions are being forged through novel and imaginative collaborations between data scientists and machine learning experts.
Rising to this challenge is digital laboratory tools provider eLabNext, which is at the forefront of creating an agnostic platform through which scientists can utilize multiple AI technologies in life science R&D. We spoke with Founder and Managing Director Erwin Seinen and his colleague Zareh Zurabyan, together with Taylor Chartier and Vadim Nazarov who founded AI software companies Modicus Prime and ImmunoMind respectively, to learn how such a collaboration is revolutionizing laboratory workflows and data analysis.
AI solutions to previously intractable R&D challenges
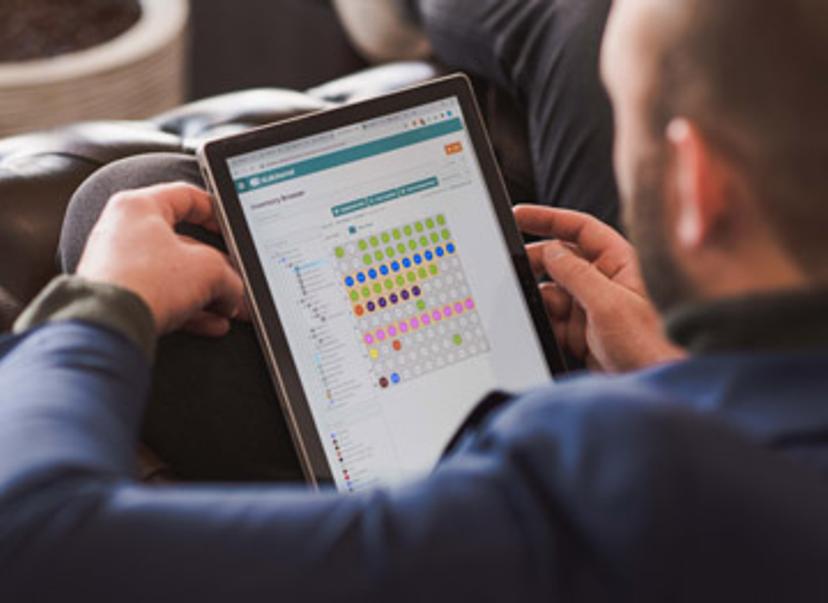
Seinen emphasizes how much science revolves around a core of consistent, reproducible results, an increasingly difficult feat with digitally assisted big data generation becoming the norm in many fields. But AI might just provide the means of alleviating current bottlenecks as Seinen explains, “AI provides the tools necessary for an equally accelerated analysis and experimental design that upholds the standards that we have lived by to this date. AI applications will help us take the inevitable next step in science”.
His colleague, Zurabyan, Head of eLabNext, Americas, division of Eppendorf, shares his enthusiasm, “I am very excited about this new age of life science and biotechnology. With Eppendorf and eLabNext, we are right at the forefront of this development, aiding the research of therapeutics that will assist you and me to live healthier and longer lives,” Zurabyan adds.
That enthusiasm is borne in part from the fruits of a highly innovative collaboration with two start-up AI software companies – Modicus Prime, which harnesses AI in image processing for particle characterization and anomaly detection, and ImmunoMind, which links immune cell function to manufacturing and treatment success in biopharmaceuticals. eLabNext has integrated both these technologies into its extensions.
Understanding and adopting new AI technologies
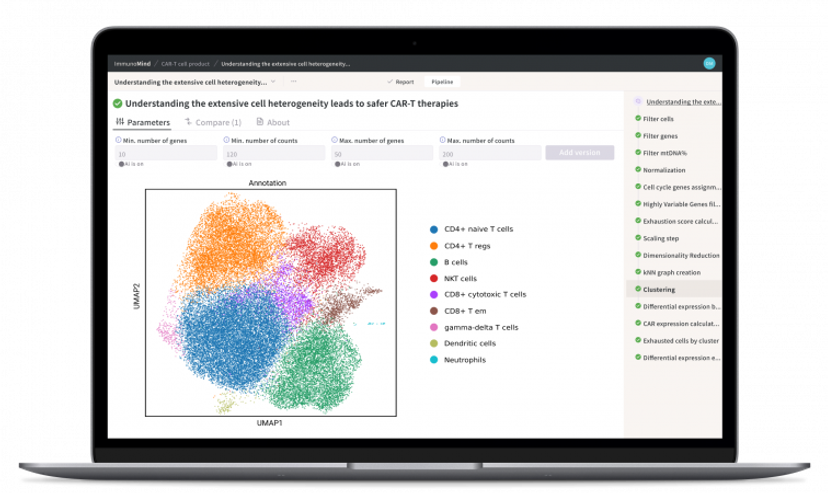
Vadim Nazarov, founder of ImmunoMind and an expert in bioinformatics and machine learning, attempts to ‘demystify’ AI and clarify exactly what it is. “AI technologies mark a paradigm shift in everything,” he says, “from how we think about using AI to solve practical tasks and make decisions using AI recommendations, to how we develop and test AI software.”
He emphasizes that AI is about writing software that ‘learns from data for itself’ to solve a specific task. “In some sense, it's about setting an environment for learning rather than just writing an algorithm,” Nazarov adds. But the paradigm shift does not end here, it goes beyond software, to education, culture, organizational processes, and even marketing.
Taylor Chartier, founder of Modicus Prime and a former engineer and data scientist from the pharmaceutical industry, expands this perspective further. “The misunderstanding that accompanies AI in the life sciences community lies predominantly in the way it has been evangelized,” she suggests. “It can simply be described as a model to better predict and interpret experimental results in the lab. What makes AI models particularly powerful is their non-linear functions interspersed with multiple layers of linear operations, giving these models the capacity to solve any given problem with an adequate number of parameters,” she further adds.
Both Chartier and Nazarov understand the benefits of adopting AI technologies in the healthcare sector, as Chartier explains, “From early-stage toxicology studies to bioprocessing scale-up, scientists can take advantage of AI by integrating the tools directly into their daily workflows. This can be done with a digital laboratory platform or lab-centralized cloud-based technology,” she says.
Chartier indicates that early adopters will realize the benefits of hybrid modeling within their experimental designs, predicting that by 2030, research within drug development will be done mostly in silico and validated with laboratory evidence. Nazarov adds that it is crucial to be an early adopter, or to work with others who are pioneering a new area. “Early adopters set the future market trends, they learn what works and what doesn't and build internal expertise and culture from trial-and-error. This is simply impossible to learn in any other environment,” he asserts.
Practicalities and current limitations in using AI
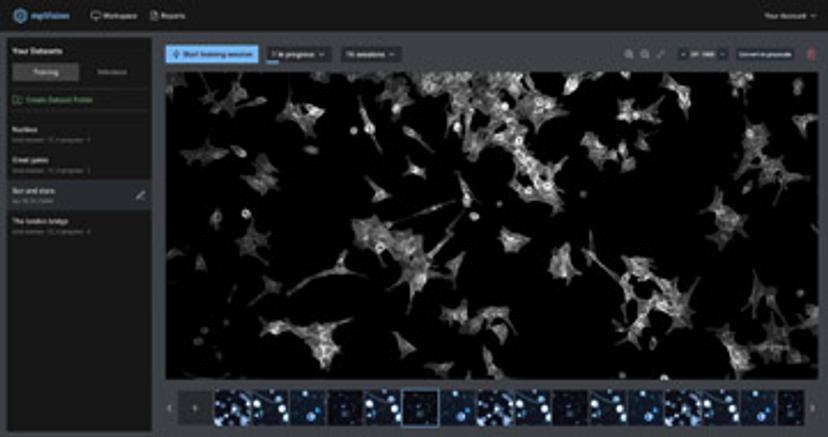
According to Nazarov, introducing AI technology is mainly about education, bringing in new expertise, and removing the mystery. Working with an external expert partner can also help. He suggests that more detailed recommendations depend on the structure of the team. For example, for more tech-oriented teams, it is important to give freedom so people can experiment with new technologies. For more biomedical-oriented teams, it is important to talk about specific results and the feedback loops from AI to team members. “It helps to show people the deliverables from AI and to build trust in the technology,” he says.
With building trust very much in mind, Chartier suggests that the final step in introducing any new AI technology is to construct a well-defined case study with the tool to demonstrate quantifiable added value to the team, such as efficiency or prediction metrics.
Chartier and Nazarov point to three main hurdles currently limiting the successful adoption of AI technologies – data, company culture, and regulation. Even the best AI algorithms will not work if data is either of poor quality or in short supply. In terms of regulation, Chartier explains that regulatory bodies including the FDA are currently still piloting AI/machine learning programs to provide better guidance for manufacturers of Software as a Medical Device (SAMD). She cites the Pre-Cert program which is still in the pilot phase with nine participating manufacturers, while adding that the FDA is doing an excellent job surveying industry and AI experts to publish guidance around trustworthy AI.
Unusual applications and future potential
Nazarov explains that AI can help to find new biomarkers for patient stratification, help develop diagnostics, or help correct errors in medical prescriptions to make them safer for patients. “In the end, it's all about bringing different perspectives to work together on better instruments because we all have the same goal of improving patient lives,” he concludes.
Chartier cites neuromorphic computing as an exciting approach that provides autonomous AI solutions with adaptability in real time, suggesting that such applications within robotics will continue to improve patients’ lives. She concludes with the following observation, “In the context of biotech, only 5% of the roughly 7,000 rare diseases have an FDA-approved drug, leaving thousands of conditions without a cure. With the help of AI augmenting researchers’ domain expertise, biotechs have the opportunity to systematically address the unmet health needs of millions.”
In his closing statement, Zurabyan at eLabNext believes that “the early adopters will bury their competition in this quest for new innovations and therapeutics. Having the ability to analyze millions of data-points at a much faster pace than those that do not use AI technologies, means scientists can search less and do more true research,” he says.
Learn more about eLabNext and how Modicus Prime and ImmunoMind AI software could help you.
Visit our new special feature to find out more about the latest advancements in digital transformation.