Standardizing metabolomics advances biomarker discovery
Discover how prioritizing reproducibility and method validation can empower researchers to unlock the full potential of metabolomics in advancing biomarker discovery and drug development
23 May 2025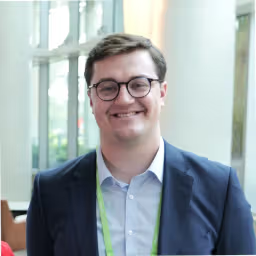
Metabolomics – the study of metabolites in cells, tissues, and body fluids – is a fast-growing area that plays a significant role in the study of health and disease and in biomarker and drug target identification. Reproducibility of results is vital, but there is a lack of widely accepted and applied standard methods for sample preparation in metabolomics. For the field to progress, further research on method development is needed to standardize sample preparation and data processing methods.
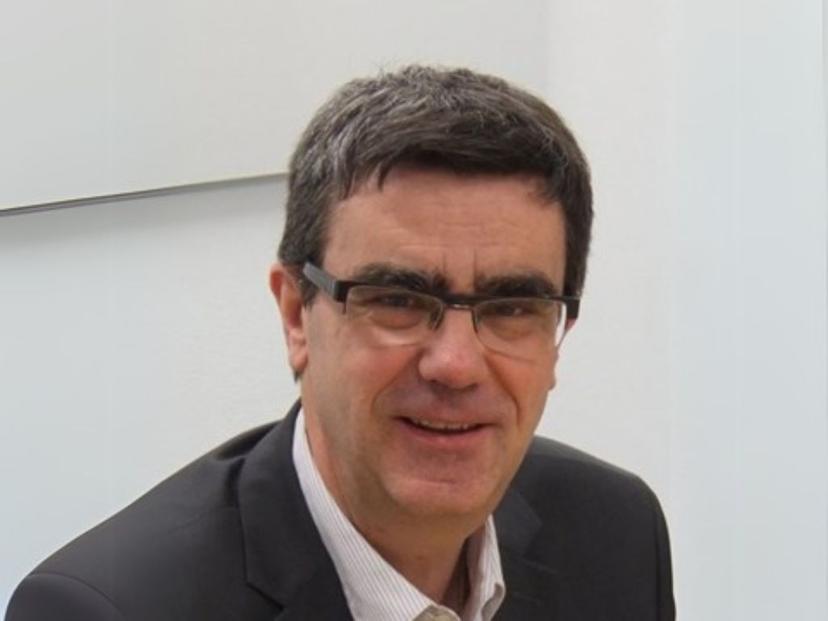
Gérard Hopfgartner is a Professor in the Department of Inorganic and Analytical Chemistry at the University of Geneva
Gérard Hopfgartner is a Professor in the Department of Inorganic and Analytical Chemistry at the University of Geneva. Hopfgartner heads up the Life Sciences Mass Spectrometry Group, a team working in analytical research based on separation science and an understanding of the importance of standardization. He explains, “Our research uses innovative technologies in metabolomics and lipidomics. In order to produce consistent and verifiable results, we focus on software and workflow in separation science and mass spectrometry for analyzing metabolites, pharmaceuticals, pesticides, lipids, and glycopeptides.”
Solving the challenges of method transfer
Methods need to be transferred from one laboratory to another to check results from an experiment or to apply techniques to other areas of research. Different operators and equipment can risk the results not being comparable. For example, in mass spectrometry (MS), electrospray ionization (ESI) is required to generate ions. If the analytical method is not fully optimized, ion suppression or enhancement effects from endogenous material in biological samples and other operating conditions and factors can affect the results. A reliable method is one that is platform-independent and can be transferred to other platforms and laboratories with the same results.
“What you do using a certain system – for example, online solid phase extraction – should be able to be transferred to other systems with the same results. We also need to be more critical of what we generate. Rather than just showing a method that has solved a specific problem, especially in the field of metabolomics, we need to focus on standardization and reproducibility across researchers and across laboratories,” says Hopfgartner. “If your method requires very high levels of technical skills, is difficult to carry out, or is very sensitive to change, it may not be the best method.”
Using the simplest possible standardized techniques and methods for sample preparation and analysis, based on an in-depth understanding of the impact of different parameters, the effect of different approaches, as well as clear written methodologies, will help to reduce or eliminate method transfer issues.
Regulatory bodies have recommended method characterization and standard operating protocols for bioanalysis in metabolomics, including the Food & Drugs Administration (FDA) and the European Medicines Agency (EMA). Working groups in different research associations have also made efforts to standardize methods. Hopfgartner believes taking into account the needs and constrains of scientists (fit-of-purpose) involved in the field is a better approach.
“Guidelines need to define the method objective and constraints, as the performance criteria will change depending on the scientific question you have to address,” says Hopfgartner.
Guidelines need to define the method objective and constraints, as the performance criteria will change depending on the scientific question you have to address.
Gérard Hopfgartner Professor in the Department of Inorganic and Analytical Chemistry at the University of Geneva
Building in reliability by focusing on sample preparation and analysis
Researchers in metabolomics use different types of analyses, dependent on the aim of the study. Targeted analysis focuses on specific metabolites, whereas untargeted analysis looks at all the sample’s detectable metabolites (known and unknown). In untargeted analysis, the sample preparation must be as minimal as possible to avoid losing analytes. Still, components such as protein in plasma and urine can impact the results and contaminate instruments. In targeted analysis, the sample can be purified to reduce interference because the metabolites are known.
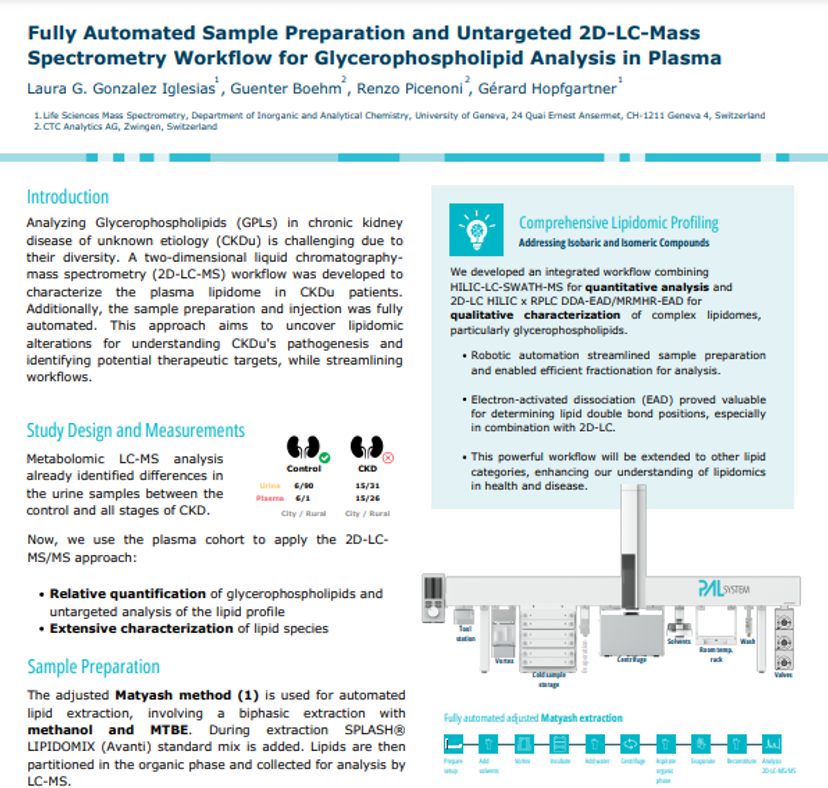
Download this poster to discover a fully automated sample preparation and untargeted 2D-LC-mass spectrometry workflow for glycerophospholipid analysis in plasma developed by Gérard Hopfgartner and colleagues
High-throughput targeted analytics rely on robustness, comparability, and reproducibility. Automation in sample preparation is an effective way to reduce variability, particularly from operator error. Hopfgartner’s team uses an autosampler with robotic capability that can be customized to their specific needs, for example, to carry out sample preparation or injection. Their setup also allows them to automate batches or single samples.
“For preparation, we prefer to work sample by sample. When coupled with chromatography, we can analyze an extract while the next sample is being processed. This means that if there is any issue in the analytical sequence and it stops, we don't lose the sample that has already been prepared. This is especially useful when carrying out untargeted analyses,” says Hopfgartner.
The chromatography analysis steps can be automated and integrated into the process. The team uses online solid phase extraction and column switching. Another approach, multi-dimensional chromatography, means that the researchers can fractionate the sample depending on the chemical property, for example, coupling hilic separation with reverse phase chromatography.
“Another technique we use is supercritical-fluid extraction and supercritical-fluid chromatography. Carbon dioxide is, to some extent at least, a green solvent, and as it evaporates, we have less solvent waste to deal with,” says Hopfgartner.
Another technique we use is supercritical-fluid extraction and supercritical-fluid chromatography. Carbon dioxide is, to some extent at least, a green solvent, and as it evaporates, we have less solvent waste to deal with.
Gérard Hopfgartner Professor in the Department of Inorganic and Analytical Chemistry at the University of Geneva
The role of quality control
While the choices for automation and standardization will vary between laboratories based on individual environments and constraints, the focus needs to remain on the quality of the data generated. This is something that is often neglected, according to Hopfgartner and needs to be allied to the method rather than an individual problem or study.
“Quality control samples are important to check the performance of the assay data, but there is a lack of a quality control step in many methods under development,” says Hopfgartner. “We need to teach the people who develop the method that they need to provide data to demonstrate performance and validate the assay.”
The future of metabolomics
One of the roles of metabolomics is to identify and analyze biomarkers. These are used to create a targeted drug, diagnose disease, and monitor its process. Biomarkers can also find the patients most likely to respond to a specific medication or least likely to experience adverse effects. As part of the drug and diagnostic approval process, pharmaceutical companies have to provide data to the regulatory authorities. This data, however, may be based on metabolic assays that have not been validated by independent authorities.
“The number of analytes and the complexity of the field is so large. If we want to use biomarkers in a clinical environment, we need to consider independent evaluation and cross-validation of the methods to ensure that we are not generating false positives and false negatives,” says Hopfgartner. “This concern for method development and validation is one of the future challenges.”