Overcoming large 3D microscopy imaging data with AI-based image analysis algorithms
Learn how a team of researchers at the Leibniz-Institut für Analytische Wissenschaften (ISAS) is developing scalable, AI-based biomedical image analysis algorithms to simplify the analysis of large image datasets
19 Jun 2022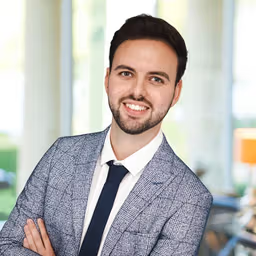
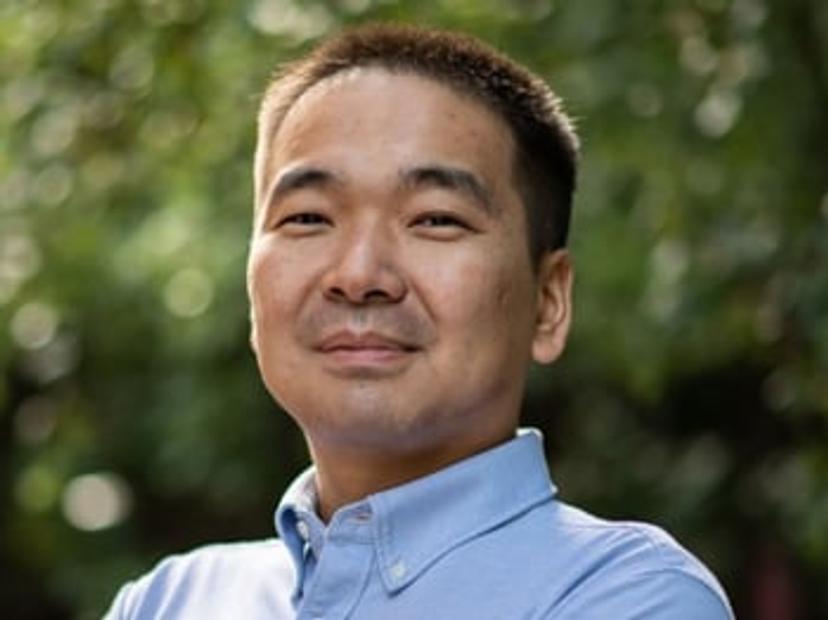
Medical imaging generates an enormous amount of data that is impossible to manually analyze. With advances in artificial intelligence (AI) researchers are now looking at how this technology can be used to help manage and simplify the analysis of large 3D microscopy image datasets.
In this SelectScience® article, we speak with Jianxu Chen, head of the new Analysis of Microscopic BIOMedical Images (AMBIOM*) group at ISAS. Chen discusses how his group is developing scalable, AI-based image analysis algorithms to help support disease studies. Chen also explores some current trends in laboratories adopting AI and machine learning.
What are the goals of your research in medical image analysis?
JC: My team is focused on developing scalable, AI-based, biomedical image analysis algorithms, especially for very large 3D microscopic image datasets. My passion is to build the ‘eyes and brains’ for computers to be able to understand large image data in biomedical studies. My team's goal is to develop new open-source methods and tools to allow broad-based new studies on the development of disease.
What are the applications of your work and how do you hope it will impact healthcare?
JC: We hope that our AI-based analysis methods will support doctors when they make diagnostic or therapeutic decisions. Among all the types of data that we are trying to develop, microscopy is one of the many application fields where AI has revolutionized the processing of enormous amounts of data. Despite existing computer programs for image evaluation and processing, our human brain is almost at its limit when it comes to dealing with such big biomedical data. That’s why our group is creating these algorithms to serve as the ‘eyes and brains’ for artificial intelligence machines to be able to analyze large image datasets.
For example, thinking about microscopy, one sample could produce over 500 images under the microscope, and this is a huge amount. For people, it will take years to manually analyze all this data. That’s why we are developing this AI, to exploit the power of this big data. In our recent work we analyzed the image of vascular structures from eight different organs in mice, we then looked at the images under the microscope and ran this through our method to quantify the vascular structure. This will provide us with the foundation for understanding the vascular structure of different organs. And in the future, I hope this will serve as a tool for biomedical researchers when they need to study vascular disease.
Are there any technologies that have helped you overcome challenges in your research?
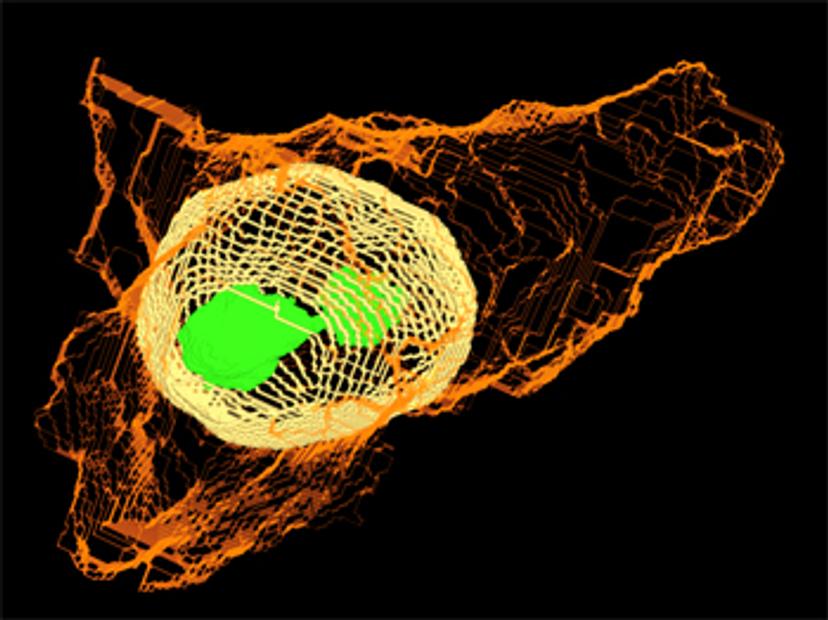
JC: At ISAS, we have a large Graphics Processing Unit (GPU) cluster. With the tremendous support from our central IT team, we set up the GPU computing cluster, and a large storage system in a reliable way. This enables us to access our data, in the office, or even at home, and this makes our research easy in terms of accessibility. All our analyses and tools that we develop should be fully reproducible and should be fully open source. It's therefore very important for us at ISAS that scientists all around the world have access to the AI methods that we develop here in Dortmund.
I think one of the biggest challenges, not only in our institute but for a lot of places where people conduct interdisciplinary research is communication. Because we are trying to bring the effort from chemists, biologists, physicists, and computer scientists, all together to solve some big problems, it's very important to establish effective communication so that people with different expertise can understand each other.
What trends have you seen in laboratories adopting AI and machine learning approaches?
JC: We have seen that studies are being conducted on a much larger scale, enabled by the new AI methods. We are also seeing studies now being carried out in tandem.
One example is trying to understand a single cell. Single cells can exist in many different forms, if you only have 10 different cells to study you only see 10 different variations and this may not show the full picture. If you have 10 million cells to study, then you will have a much better understanding of what is going on. There are now new possibilities to study large amounts of cells, and this is enabled by AI. There is now the possibility of taking several thousands of microscopic images of cells, analyzing them, and generating a very holistic view of several millions of cells.
Could you tell us about the session that you are chairing at analytica 2022? What can we look forward to?
JC: I'm excited to have the chance to introduce four talks at analytica 2022 and will be chairing the Research Data Management I: AI for Image Analysis session. The four speakers for the session are from very different subfields of the overall AI-based research field. Dr. Dagmar Kainmueller, Max Delbrück Center for Molecular Medicine (MDC), will discuss automated cell segmentation classification and tracking in large-scale microscopic data. This is an exciting topic as we need AI-based methods to help us understand cells with higher accuracy and at a large scale.
Prof. Dr. Marc Aubreville, Technische Hochschule Ingolstadt (THI), will discuss large datasets in AI. We know that AI requires a lot of data, and for clinical or biomedical applications, getting a large set of data from humans is not easy. The issues, challenges, and opportunities in AI for these applications will be covered in this talk.
Prof. Dr. Yiyu Shi, University of Notre Dame, will introduce some of his work in using deep learning for congenital heart disease modeling and diagnosis. Shi will also discuss how an AI algorithm enabled the very first telemonitoring of heart surgery.
The last talk will be presented by Michael Baumgartner, Deutsches Krebsforschungszentrum. Baumgartner will introduce a new method where AI researchers don’t need to manually configure methods. There are lots of hyper-parameters that people must tweak when designing a method. They must set how fast you should learn, and which model you should use, and many people don't know how to choose. Now we can teach the machine to choose these parameters for us.
As you can see the talks are all from very different subfields and will give deep insight into the very cutting edge of AI research for analytical science.
*AMBIOM is funded by the German Federal Ministry of Education and Research.